Fine-Tuning vs Frontier Models: Making the Right AI Investment
April 14, 2025 | Ameya Kanitkar, CTO
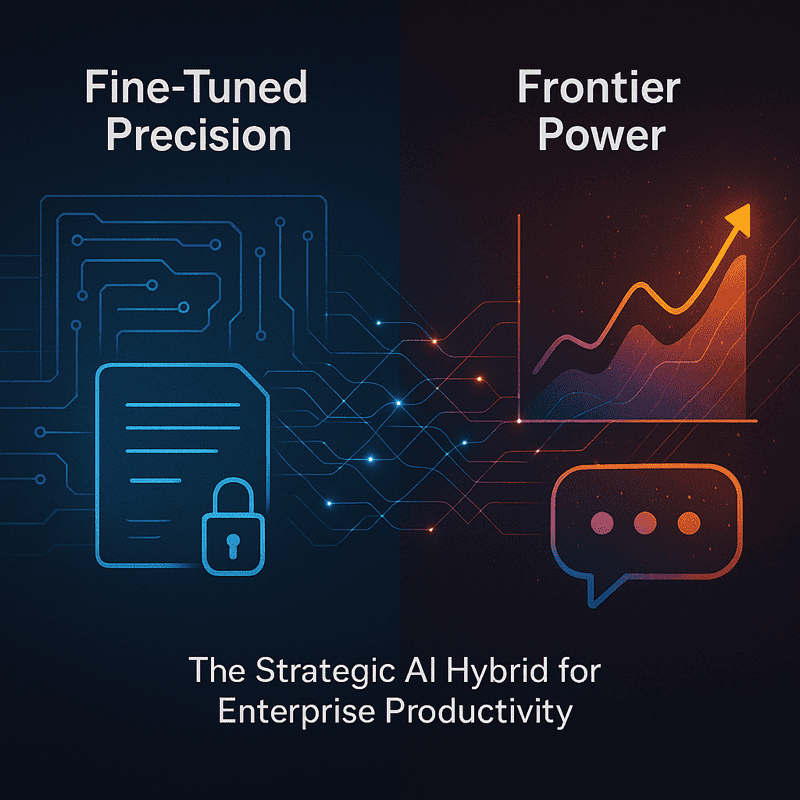
At Larridin, we focus on helping organizations understand and improve knowledge work productivity. However, measuring real productivity isn’t straightforward. Common metrics like lines of code or emails sent can mislead—often rewarding quantity over quality. True productivity insights emerge from subtle interactions: how engineers discuss complex problems, how salespeople navigate intricate negotiations, or how efficiently operations teams overcome challenges. Capturing these nuanced signals demands AI that truly understands context.
At Larridin, we initially invested heavily in fine-tuning models using Low-Rank Adaptation (LoRA). These specialized models effectively picked up domain-specific nuances, delivering solid results early on.
However, AI moves at lightning speed. In recent weeks, frontier models like Gemini 2.5, GPT-4.5, and Claude Sonnet 3.7 have advanced dramatically. With carefully crafted prompts, these models outperform our finely-tuned LoRA solutions significantly.
This rapid evolution presents a strategic question: Should organizations continue to invest in fine-tuning specialized models, or leverage the raw power of frontier LLMs?
Fine-Tuning (LoRA + RAG): Specialized Precision
In fine-tuning, small adapter layers are trained atop large base models, optimizing them for specific tasks without retraining the entire model.
Advantages:
- Data Privacy & Security: Models run locally, keeping sensitive data securely contained.
- Cost Efficiency & Speed: Lean models ensure lower costs and rapid inference, ideal for real-time use cases.
- Deep Domain Expertise: Highly accurate for specialized tasks and organizational contexts.
Challenges:
- Resource Intensive: Requires sustained investment in MLOps infrastructure, data curation, and model management.
Frontier Models (Large LLMs + Prompt Engineering): Broad Excellence
Alternatively, organizations can directly leverage advanced, general-purpose models, utilizing detailed prompting to guide their reasoning.
Advantages:
- Advanced Reasoning: Immediate access to cutting-edge AI capabilities.
- Rapid Deployment: Crafting effective prompts typically outpaces full model fine-tuning.
Challenges:
- Privacy Concerns: Data typically processed externally, requiring rigorous anonymization and compliance measures.
- Higher Costs & Latency: API-driven inference can be expensive and slower.
- Prompt Expertise Needed: Achieving optimal results demands expert-level prompting.
Our Hybrid Approach at Larridin
At Larridin, we’ve adopted a strategic hybrid model:
We use Frontier Models when:
- Complex, generalized reasoning capabilities are crucial.
- Data privacy can be effectively managed through anonymization.
- Premium insights justify additional inference costs.
We deploy Fine-Tuned LoRA Models when:
- Strict privacy, regulatory compliance (GDPR, HIPAA), or local deployment are essential.
- Efficiency and scalability at lower costs are critical.
- Specialized tasks require deep understanding of domain-specific nuances.
Frequently, fine-tuned models handle initial data processing—such as filtering or anonymizing, classification—before leveraging frontier models for deeper analysis. We continuously evaluate our approach to adapt to evolving AI capabilities.
Privacy and Ethics: Non-negotiable
Our commitment is clear: analyzing productivity trends and organizational effectiveness—not individual monitoring. We maintain rigorous standards of privacy and ethical data handling regardless of the AI techniques deployed.
Final Thought
Selecting between fine-tuned models and frontier LLMs isn’t just a technical choice; it’s a strategic investment decision. By employing a balanced hybrid approach, we ensure our clients receive precise, actionable insights tailored exactly to their needs, also allowing us to take advantage of future developments.
P.S. At the time of writing, LLMA4 has just been released along with LLMA4 Scout—a smaller, faster model featuring a 10M token context window. This recent development further validates our hybrid approach